Projects
Thesis
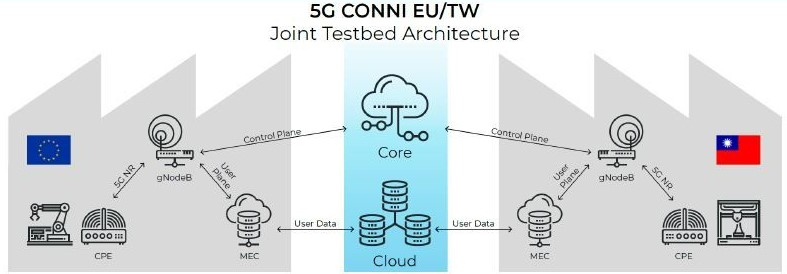
[T] Centralised orchestration and hybrid resource management for Ultra Reliable and Low Latency Communications (URLLC)
5G Communications, Hybrid Resource Management, Lyapunov Optimization, Multi-Agent Reinforcement Learning, System Level Simulation.
Abstract Relying on Lyapunov’s optimizations for two-queue state system management, we design an optimization framework in which RAN latency, reliability and resource efficiency are considered. Afterwards, we implement an OpenAirInterface (OAI) testbed for the validation of our algorithms. This implementation step is a proof of their feasibility under real time restrictions, and this step illustrates performance of our algorithms in experimentation. Finally, we propose a novel hybrid Grant-Based (GB) and Grant-Free (GF) radio access scheme using Multi Agent Reinforcement Learning (MARL) for URLLC. Link
5G Communications, Hybrid Resource Management, Lyapunov Optimization, Multi-Agent Reinforcement Learning, System Level Simulation.
Abstract Relying on Lyapunov’s optimizations for two-queue state system management, we design an optimization framework in which RAN latency, reliability and resource efficiency are considered. Afterwards, we implement an OpenAirInterface (OAI) testbed for the validation of our algorithms. This implementation step is a proof of their feasibility under real time restrictions, and this step illustrates performance of our algorithms in experimentation. Finally, we propose a novel hybrid Grant-Based (GB) and Grant-Free (GF) radio access scheme using Multi Agent Reinforcement Learning (MARL) for URLLC. Link
Patents
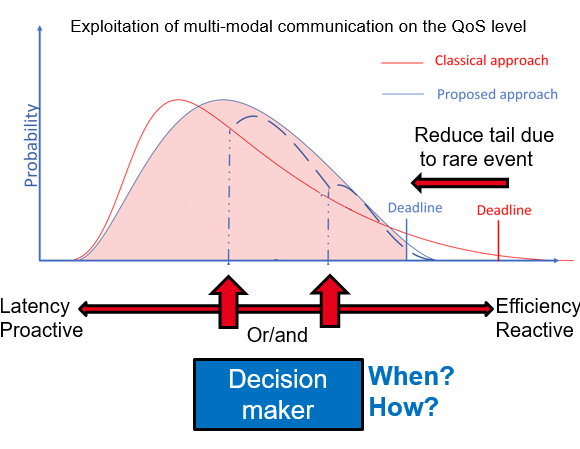
[P] Method to exploit latency distribution for early decision making, Patent 2103542, filled in March,2021
Early Decision Making.
Abstract The invention is concerned generally with wireless networks and in particular with a method and apparatus for orchestrating the execution of a plurality of mechanisms by one or more nodes in a wireless network. Link
Early Decision Making.
Abstract The invention is concerned generally with wireless networks and in particular with a method and apparatus for orchestrating the execution of a plurality of mechanisms by one or more nodes in a wireless network. Link
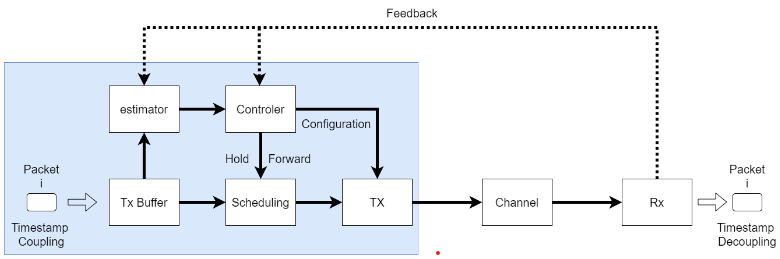
[P] Methods and apparatus for jitter-aware scheduling in wireless Time Sensitive Network communications, Patent 2103542, filled in May,2021
Network Determinism.
Abstract We introduce a jitter-aware orchestration method that forces latency to fall within predetermined windows. Link
Network Determinism.
Abstract We introduce a jitter-aware orchestration method that forces latency to fall within predetermined windows. Link
Journal articles
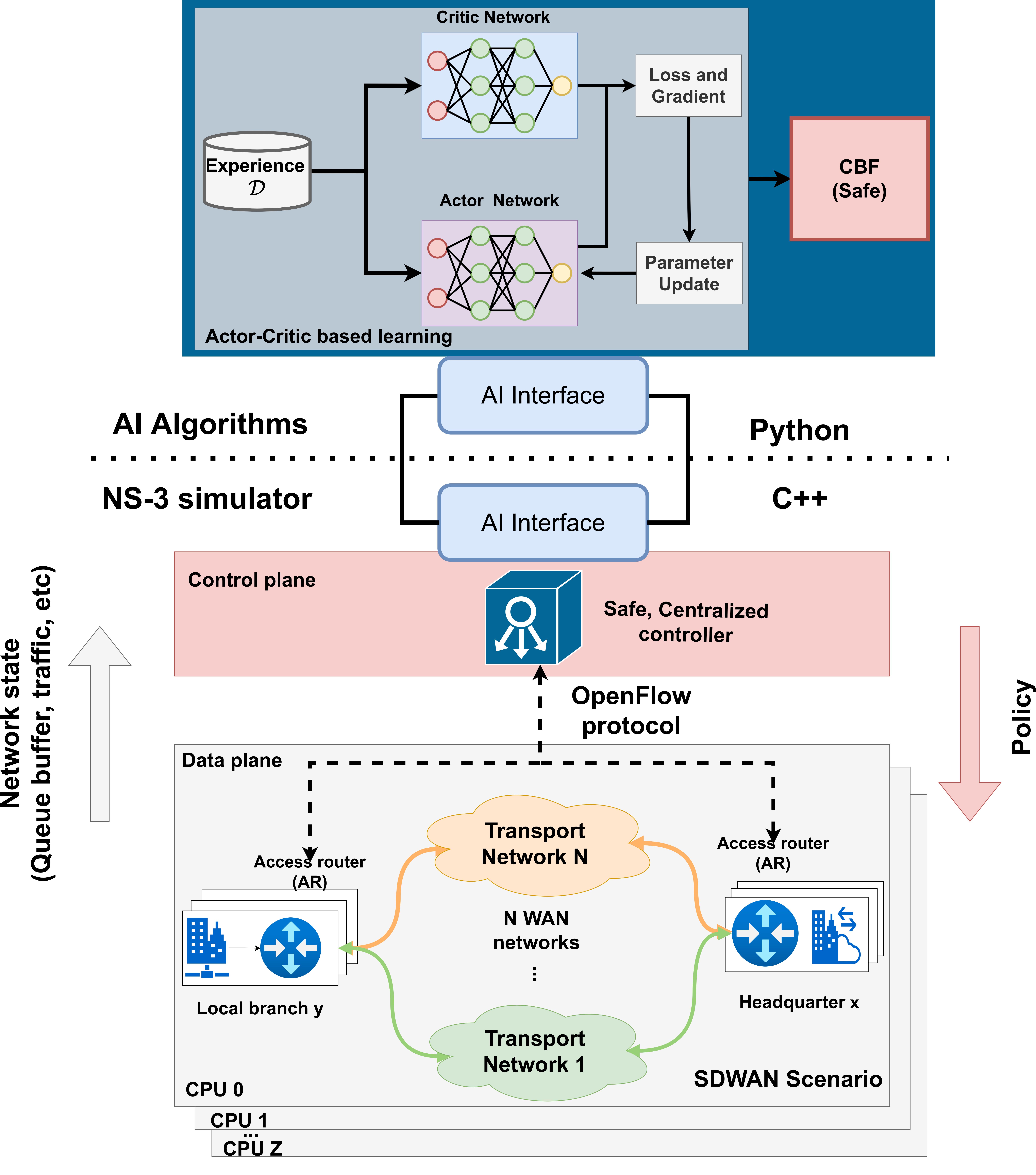
[J] Safe Load Balancing in Software-Defined-Networking
Traffic Engineering, Optimisation, Deep Reinforcement Learning, Heuristic, Transfer Learning, GPU acceleration.
Abstract High performance, reliability and safety are crucial properties of any Software-Defined-Networking (SDN) system. Although the use of Deep Reinforcement Learning (DRL) algorithms has been widely studied to improve performance, their practical applications are still limited as they fail to ensure safe operations in exploration and decision-making. To fill this gap, we explore the design of a Control Barrier Function (CBF) on top of Deep Reinforcement Learning (DRL) algorithms for load-balancing. We show that our DRL-CBF approach is capable of meeting safety requirements during training and testing while achieving near-optimal performance in testing. We provide results using two simulators: a flow-based simulator, which is used for proof-of-concept and benchmarking, and a packet-based simulator that implements real protocols and scheduling. Thanks to the flow-based simulator, we compared the performance against the optimal policy, solving a Non Linear Programming (NLP) problem with the SCIP solver. Furthermore, we showed that pre-trained models in the flow-based simulator, which is faster, can be transferred to the packet simulator, which is slower but more accurate, with some fine-tuning. Overall, the results suggest that near-optimal Quality-of-Service (QoS) performance in terms of end-to-end delay can be achieved while safety requirements related to link capacity constraints are guaranteed. In the packet-based simulator, we also show that our DRL-CBF algorithms outperform non-RL baseline algorithms. When the models are fine-tuned over a few episodes, we achieved smoother QoS and safety in training, and similar performance in testing compared to the case where models have been trained from scratch. Link
Traffic Engineering, Optimisation, Deep Reinforcement Learning, Heuristic, Transfer Learning, GPU acceleration.
Abstract High performance, reliability and safety are crucial properties of any Software-Defined-Networking (SDN) system. Although the use of Deep Reinforcement Learning (DRL) algorithms has been widely studied to improve performance, their practical applications are still limited as they fail to ensure safe operations in exploration and decision-making. To fill this gap, we explore the design of a Control Barrier Function (CBF) on top of Deep Reinforcement Learning (DRL) algorithms for load-balancing. We show that our DRL-CBF approach is capable of meeting safety requirements during training and testing while achieving near-optimal performance in testing. We provide results using two simulators: a flow-based simulator, which is used for proof-of-concept and benchmarking, and a packet-based simulator that implements real protocols and scheduling. Thanks to the flow-based simulator, we compared the performance against the optimal policy, solving a Non Linear Programming (NLP) problem with the SCIP solver. Furthermore, we showed that pre-trained models in the flow-based simulator, which is faster, can be transferred to the packet simulator, which is slower but more accurate, with some fine-tuning. Overall, the results suggest that near-optimal Quality-of-Service (QoS) performance in terms of end-to-end delay can be achieved while safety requirements related to link capacity constraints are guaranteed. In the packet-based simulator, we also show that our DRL-CBF algorithms outperform non-RL baseline algorithms. When the models are fine-tuned over a few episodes, we achieved smoother QoS and safety in training, and similar performance in testing compared to the case where models have been trained from scratch. Link
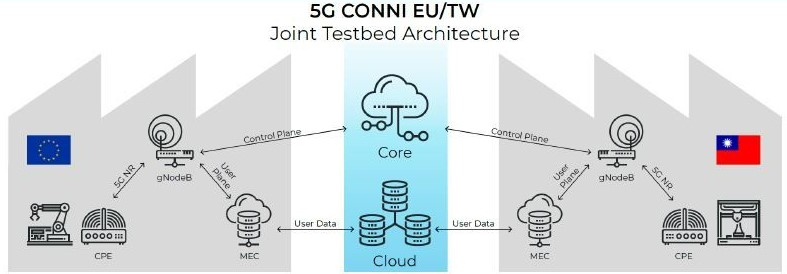
[J] Beyond Private 5G Networks: Applications, Architectures, Operator Models and Technological Enablers
5G Network Architecture and Orchestration.
Abstract Private networks will play a key role in 5G and beyond to enable smart factories with the required better deployment, operation and flexible usage of available resource and infrastructure. 5G private networks will offer a lean and agile solution to effectively deploy and operate services with stringent and heterogeneous constraints in terms of reliability, latency, re-configurability and re-deployment of resources as well as issues related to governance and ownership of 5G components, and elements. In this paper, we present a novel approach to operator models, specifically targeting 5G and beyond private networks. We apply the proposed operator models to different network architecture options and to a selection of relevant use cases offering mixed private–public network operator governance and ownership. Moreover, several key enabling technologies have been identified for 5G private networks. Before the deployment, stakeholders should consider spectrum allocation and on-site channel measurements in order to fully understand the propagation characteristic of a given environment and to set up end-to-end system parameters. During the deployment, a monitoring tools will support to validate the deployment and to make sure that the end-to-end system meet the target KPI. Finally, some optimization can be made individually for service placement, network slicing and orchestration or jointly at radio access, multi-access edge computing or core network level. Link
5G Network Architecture and Orchestration.
Abstract Private networks will play a key role in 5G and beyond to enable smart factories with the required better deployment, operation and flexible usage of available resource and infrastructure. 5G private networks will offer a lean and agile solution to effectively deploy and operate services with stringent and heterogeneous constraints in terms of reliability, latency, re-configurability and re-deployment of resources as well as issues related to governance and ownership of 5G components, and elements. In this paper, we present a novel approach to operator models, specifically targeting 5G and beyond private networks. We apply the proposed operator models to different network architecture options and to a selection of relevant use cases offering mixed private–public network operator governance and ownership. Moreover, several key enabling technologies have been identified for 5G private networks. Before the deployment, stakeholders should consider spectrum allocation and on-site channel measurements in order to fully understand the propagation characteristic of a given environment and to set up end-to-end system parameters. During the deployment, a monitoring tools will support to validate the deployment and to make sure that the end-to-end system meet the target KPI. Finally, some optimization can be made individually for service placement, network slicing and orchestration or jointly at radio access, multi-access edge computing or core network level. Link
Conference proceedings
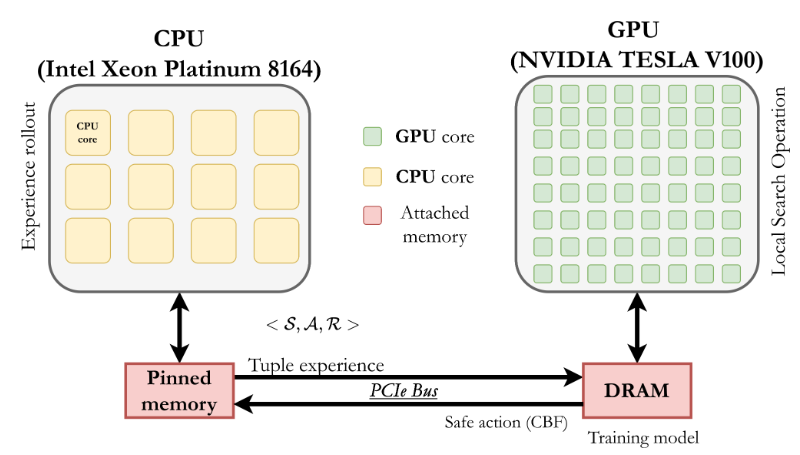
[C] Towards Safe Load Balancing based on Control Barrier Functions and Deep Reinforcement Learning
Network optimization, CUDA-enabled acceleration, Safety, Deep Reinforcement Learning (DRL), Control Barrier Function (CBF).
Abstract Deep Reinforcement Learning (DRL) algorithms have recently made significant strides in improving network performance. Nonetheless, their practical use is still limited in the absence of safe exploration and safe decision-making. In the context of commercial solutions, reliable and safe-to-operate systems are of paramount importance. Taking this problem into account, we propose a safe learning-based load balancing algorithm for Software Defined-Wide Area Network (SD-WAN), which is empowered by Deep Reinforcement Learning (DRL) combined with a Control Barrier Function (CBF). It safely projects unsafe actions into feasible ones during both training and testing, and it guides learning towards safe policies. We successfully implemented the solution on GPU to accelerate training by approximately 110x times and achieve model updates for on-policy methods within a few seconds, making the solution practical. We show that our approach delivers near-optimal Quality-of-Service (QoS) performance in terms of end-to-end delay while respecting safety requirements related to link capacity constraints. We also demonstrated that on-policy learning based on Proximal Policy Optimization (PPO) performs better than off-policy learning with Deep Deterministic Policy Gradient (DDPG) when both are combined with a CBF for safe load balancing. Link
Network optimization, CUDA-enabled acceleration, Safety, Deep Reinforcement Learning (DRL), Control Barrier Function (CBF).
Abstract Deep Reinforcement Learning (DRL) algorithms have recently made significant strides in improving network performance. Nonetheless, their practical use is still limited in the absence of safe exploration and safe decision-making. In the context of commercial solutions, reliable and safe-to-operate systems are of paramount importance. Taking this problem into account, we propose a safe learning-based load balancing algorithm for Software Defined-Wide Area Network (SD-WAN), which is empowered by Deep Reinforcement Learning (DRL) combined with a Control Barrier Function (CBF). It safely projects unsafe actions into feasible ones during both training and testing, and it guides learning towards safe policies. We successfully implemented the solution on GPU to accelerate training by approximately 110x times and achieve model updates for on-policy methods within a few seconds, making the solution practical. We show that our approach delivers near-optimal Quality-of-Service (QoS) performance in terms of end-to-end delay while respecting safety requirements related to link capacity constraints. We also demonstrated that on-policy learning based on Proximal Policy Optimization (PPO) performs better than off-policy learning with Deep Deterministic Policy Gradient (DDPG) when both are combined with a CBF for safe load balancing. Link
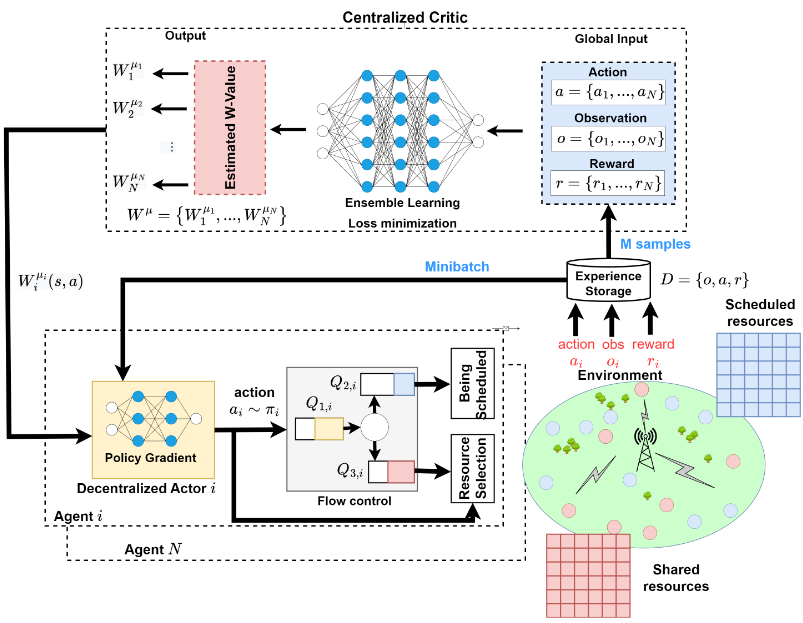
[C] Hybrid Radio Resource Management Based on Multi-Agent Reinforcement Learning
Wireless optimization, Multi-Agent Deep Reinforcement Learning (MARL), System level simulation.
Abstract In this paper, we propose a novel hybrid grant-based and grant-free radio access scheme. We provide two multi-agent reinforcement learning algorithms to optimize a global network objective in terms of latency, reliability and network throughput: Multi-Agent Deep Q-Learning (MADQL) and Multi-Agent Deep Deterministic Policy Gradient (MADDPG). In MADQL, each user (agent) learns its optimal action-value function, which is based only on its local observation, and performs an optimal opportunistic action using the shared spectrum. MADDPG involves the attached gNB function as a global observer (critic), which criticizes the action of each associated agent (actor) in the network. By leveraging centralised training and decentralised execution, we achieve a shared goal better than the first algorithm. Then, through a system level simulation where the full protocol stack is considered, we show the gain of our approach to efficiently manage radio resources and guarantee latency. Link
Wireless optimization, Multi-Agent Deep Reinforcement Learning (MARL), System level simulation.
Abstract In this paper, we propose a novel hybrid grant-based and grant-free radio access scheme. We provide two multi-agent reinforcement learning algorithms to optimize a global network objective in terms of latency, reliability and network throughput: Multi-Agent Deep Q-Learning (MADQL) and Multi-Agent Deep Deterministic Policy Gradient (MADDPG). In MADQL, each user (agent) learns its optimal action-value function, which is based only on its local observation, and performs an optimal opportunistic action using the shared spectrum. MADDPG involves the attached gNB function as a global observer (critic), which criticizes the action of each associated agent (actor) in the network. By leveraging centralised training and decentralised execution, we achieve a shared goal better than the first algorithm. Then, through a system level simulation where the full protocol stack is considered, we show the gain of our approach to efficiently manage radio resources and guarantee latency. Link
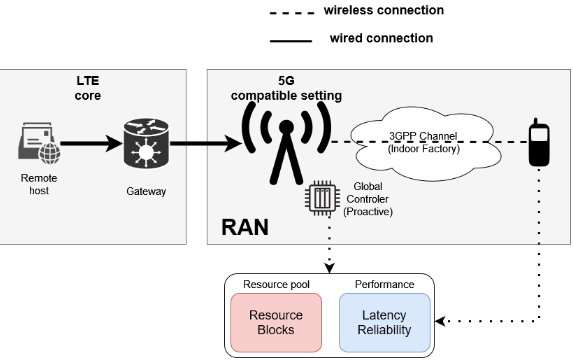
[C] Dynamic Resource Scheduling Optimization for Ultra-Reliable Low Latency Communications: From Simulation to Experimentation
Lyapunov optimization, System level simulation, Hardware experimentation.
Abstract In this paper, we propose a dynamic and efficient resource scheduling based on Lyapunov's optimization for Ultra-Reliable Low Latency Communications, taking into account the traffic arrival at the network layer, the queue behaviors at the data link layer and the risk that the applied decision might result in packet losses. The trade-off between the resource efficiency, latency and reliability is achieved by the timing and intensity of decisions and is adapted to dynamic scenarios (e.g., random bursty traffic, time-varying channel). Our queue-aware and channel-aware solution is evaluated in terms of latency, reliability outage and resource efficiency in a system-level simulator and validated by an experimental testbed using OpenAirInterface. Link
Lyapunov optimization, System level simulation, Hardware experimentation.
Abstract In this paper, we propose a dynamic and efficient resource scheduling based on Lyapunov's optimization for Ultra-Reliable Low Latency Communications, taking into account the traffic arrival at the network layer, the queue behaviors at the data link layer and the risk that the applied decision might result in packet losses. The trade-off between the resource efficiency, latency and reliability is achieved by the timing and intensity of decisions and is adapted to dynamic scenarios (e.g., random bursty traffic, time-varying channel). Our queue-aware and channel-aware solution is evaluated in terms of latency, reliability outage and resource efficiency in a system-level simulator and validated by an experimental testbed using OpenAirInterface. Link
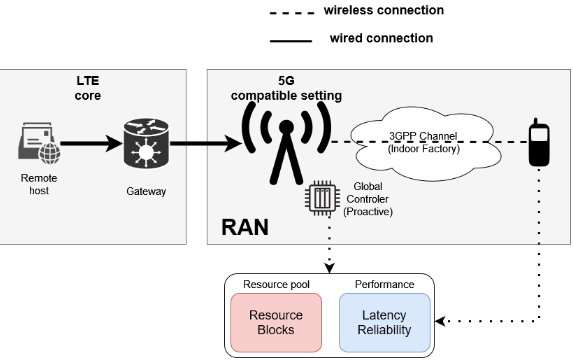
[C] Towards URLLC with Proactive HARQ Adaptation
Lyapunov optimization, System level simulation.
Abstract In this work, we propose a dynamic decision maker algorithm to improve the proactive HARQ protocol for beyond 5G networks. Based on Lyapunov stochastic optimization, our adaptation control framework dynamically selects the number of proactive retransmissions for intermittent URLLC traffic scenarios under time-varying channel conditions without requiring any prior knowledge associated with this stochastic process. It then better exploits the trade-off between Radio Access Network (RAN) latency, reliability and resource efficiency, which is still limited in its realization on current HARQ designs. We then evaluate the performance of several HARQ strategies and show that our proposal further improves latency over the reactive regime without affecting the resource efficiency such as fixed proactive retransmission while maintaining target reliability. Link
Lyapunov optimization, System level simulation.
Abstract In this work, we propose a dynamic decision maker algorithm to improve the proactive HARQ protocol for beyond 5G networks. Based on Lyapunov stochastic optimization, our adaptation control framework dynamically selects the number of proactive retransmissions for intermittent URLLC traffic scenarios under time-varying channel conditions without requiring any prior knowledge associated with this stochastic process. It then better exploits the trade-off between Radio Access Network (RAN) latency, reliability and resource efficiency, which is still limited in its realization on current HARQ designs. We then evaluate the performance of several HARQ strategies and show that our proposal further improves latency over the reactive regime without affecting the resource efficiency such as fixed proactive retransmission while maintaining target reliability. Link
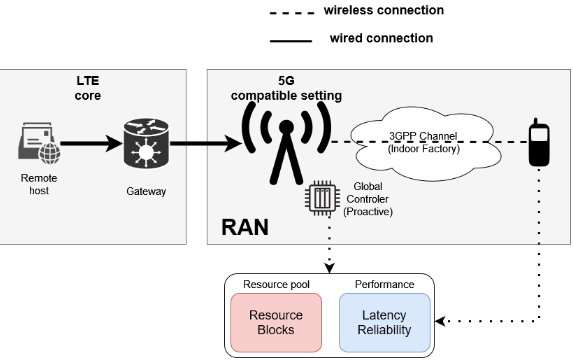
[C] Proactive Resource Scheduling for 5G and Beyond Ultra-Reliable Low Latency Communications
System level simulation.
Abstract Effective resource use in Ultra-Reliable and Low-Latency Communications (URLLC) is one of the main challenges for 5G and beyond systems. In this paper, we propose a novel scheduling methodology (combining reactive and proactive resource allocation strategies) specifically devised for URLLC services. Our ultimate objective is to characterize the level of proactivity required to cope with various scenarios. Specifically, we propose to operate at the scheduling level, addressing the trade-off between reliability, latency and resource efficiency. We offer an evaluation of the proposed methodology in the case of the well-known Hybrid Automatic Repeat reQuest (HARQ) protocol in which the proactive strategy allows a number of parallel retransmissions instead of the ‘'send-wait-react’' mode. To this end, we propose some deviations from the HARQ procedure and benchmark the performance in terms of latency, reliability outage and resource efficiency as a function of the level of proactivity. Afterwards, we highlight the critical importance of proactive adaptation in dynamic scenarios (i.e. with changing traffic rates and channel conditions). Link
System level simulation.
Abstract Effective resource use in Ultra-Reliable and Low-Latency Communications (URLLC) is one of the main challenges for 5G and beyond systems. In this paper, we propose a novel scheduling methodology (combining reactive and proactive resource allocation strategies) specifically devised for URLLC services. Our ultimate objective is to characterize the level of proactivity required to cope with various scenarios. Specifically, we propose to operate at the scheduling level, addressing the trade-off between reliability, latency and resource efficiency. We offer an evaluation of the proposed methodology in the case of the well-known Hybrid Automatic Repeat reQuest (HARQ) protocol in which the proactive strategy allows a number of parallel retransmissions instead of the ‘'send-wait-react’' mode. To this end, we propose some deviations from the HARQ procedure and benchmark the performance in terms of latency, reliability outage and resource efficiency as a function of the level of proactivity. Afterwards, we highlight the critical importance of proactive adaptation in dynamic scenarios (i.e. with changing traffic rates and channel conditions). Link
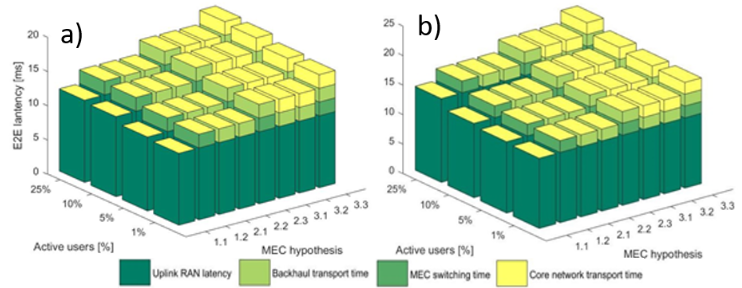
[C] Evaluation of 5G-NR V2N Connectivity in a Centralized Cooperative Lane Change Scenario
5G-NR, Vehicular Networks, System level simulation.
Abstract By means of system-level simulations, we analyze in this paper the performance of Vehicle-to-Network (V2N) connectivity based on the 5th Generation - New Radio (5GNR) as a support to Cooperative, Connected and Automated Mobility (CCAM), in light of both network and Multi-access Edge Computing (MEC) deployments. Focusing on a canonical centralized Cooperative Lane Change (CLC) use case that involves three vehicles in a cross-border highway environment, we assess the link reliability and the End-to-End (E2E) latency of all the messages involved in the CLC negotiation phase (from/to interconnected MECs hosting the centralized maneuvering application), while assuming different deployment configurations and the coexistence with a second demanding vehicular service running over the same radio resources. On this occasion, we illustrate possible benefits from Bandwidth Partitioning (BWP) on Uplink (UL) latency, as well as from an hypothetically tight cooperation between Mobile Network Operators (MNOs) on reliability and continuity, leveraging low-latency inter-MEC transactions and seamless cross-border handover capabilities. Link
5G-NR, Vehicular Networks, System level simulation.
Abstract By means of system-level simulations, we analyze in this paper the performance of Vehicle-to-Network (V2N) connectivity based on the 5th Generation - New Radio (5GNR) as a support to Cooperative, Connected and Automated Mobility (CCAM), in light of both network and Multi-access Edge Computing (MEC) deployments. Focusing on a canonical centralized Cooperative Lane Change (CLC) use case that involves three vehicles in a cross-border highway environment, we assess the link reliability and the End-to-End (E2E) latency of all the messages involved in the CLC negotiation phase (from/to interconnected MECs hosting the centralized maneuvering application), while assuming different deployment configurations and the coexistence with a second demanding vehicular service running over the same radio resources. On this occasion, we illustrate possible benefits from Bandwidth Partitioning (BWP) on Uplink (UL) latency, as well as from an hypothetically tight cooperation between Mobile Network Operators (MNOs) on reliability and continuity, leveraging low-latency inter-MEC transactions and seamless cross-border handover capabilities. Link
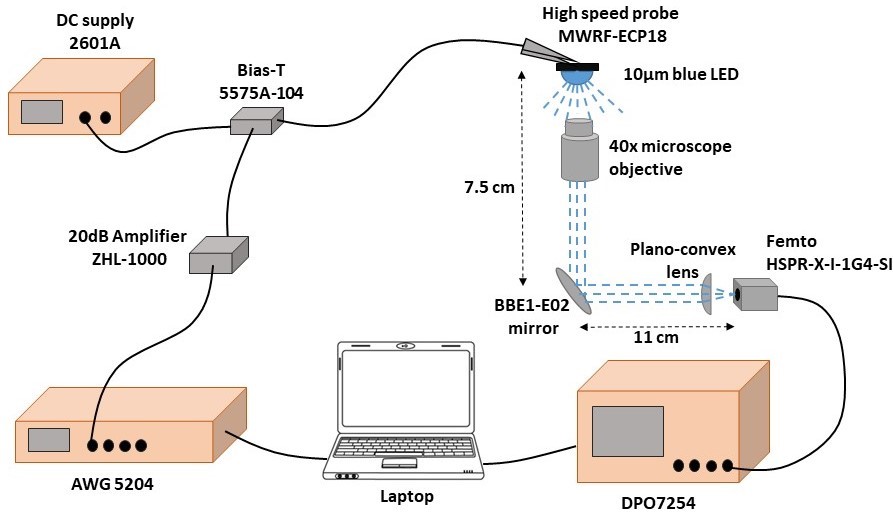
[C] Reaching 7.7 Gb/s in OWC with DCO-OFDM on a Single Blue 10-um GaN Micro-LED
Optical-Digital Signal Processing, micro-LED characterization
Abstract This presentation describes recent activities on ultra-high speed Optical Wireless Communications (OWC) using Gallium-Nitride micro-LEDs designed and fabricated at CEA-Leti. Micro-LEDs are one of the most promising OWC optical sources due to their high illumination efficiency and their large modulation bandwidths. Preliminary work focused on the implementation of a 10-µm single blue micro-LED on sapphire wafer within an experimental OWC setup, mixing software generation of direct-current optical orthogonal frequency division multiplexing (DCO-OFDM) patterns and hardware optical components for light collection, high speed photo-detection and digital acquisitions. Intensity modulation conveys DCO-OFDM waveform and direct detection is used at reception. A high current density of 25.5 kA/cm² provided a modulation bandwidth of 1.8 GHz. Associated to bit and power loading with up to a 256-QAM subcarrier modulation, it enabled a new data rate of 7.7 Gb/s, compared to the previous record of 5.37 Gb/s reached with a blue 21-µm microLED in 2016. Towards a better understanding of the micro-LED design impact on OWC performance, next investigations will study the electrical modelling of such micro-LEDs in the high frequency regime. Future works will cover the use of large arrays of more than 10 thousands micro-LEDs. The first objective is to open the way to new digital-to-optical modulations by independently driving each pixel, to remove digital-to-analogue converter and target highly integrated system-on-chips for ultra-high speed OWC transmitters. Secondly, higher emitted optical power is expected to open such technology to indoor multiple access applications where light collection and emitter-receiver alignment may not be possible anymore. Link News
Optical-Digital Signal Processing, micro-LED characterization
Abstract This presentation describes recent activities on ultra-high speed Optical Wireless Communications (OWC) using Gallium-Nitride micro-LEDs designed and fabricated at CEA-Leti. Micro-LEDs are one of the most promising OWC optical sources due to their high illumination efficiency and their large modulation bandwidths. Preliminary work focused on the implementation of a 10-µm single blue micro-LED on sapphire wafer within an experimental OWC setup, mixing software generation of direct-current optical orthogonal frequency division multiplexing (DCO-OFDM) patterns and hardware optical components for light collection, high speed photo-detection and digital acquisitions. Intensity modulation conveys DCO-OFDM waveform and direct detection is used at reception. A high current density of 25.5 kA/cm² provided a modulation bandwidth of 1.8 GHz. Associated to bit and power loading with up to a 256-QAM subcarrier modulation, it enabled a new data rate of 7.7 Gb/s, compared to the previous record of 5.37 Gb/s reached with a blue 21-µm microLED in 2016. Towards a better understanding of the micro-LED design impact on OWC performance, next investigations will study the electrical modelling of such micro-LEDs in the high frequency regime. Future works will cover the use of large arrays of more than 10 thousands micro-LEDs. The first objective is to open the way to new digital-to-optical modulations by independently driving each pixel, to remove digital-to-analogue converter and target highly integrated system-on-chips for ultra-high speed OWC transmitters. Secondly, higher emitted optical power is expected to open such technology to indoor multiple access applications where light collection and emitter-receiver alignment may not be possible anymore. Link News
Certificates
Intellectual Property
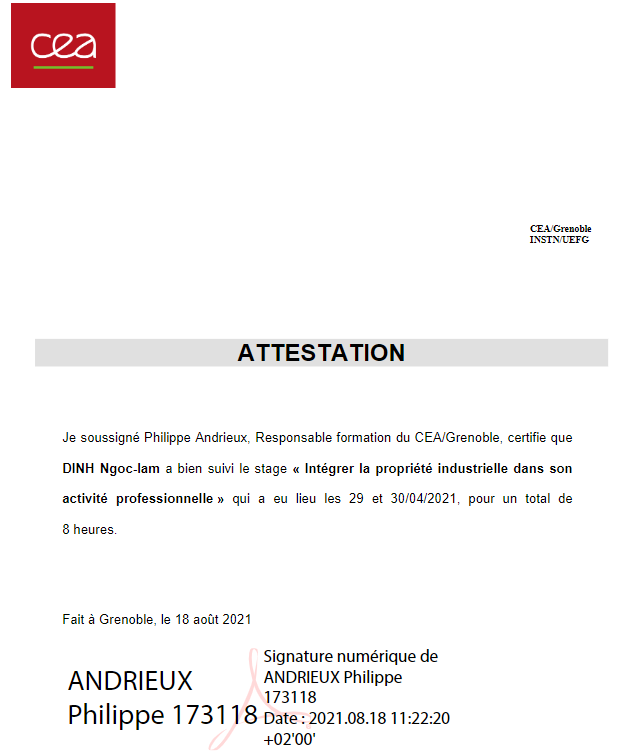
Generative AI
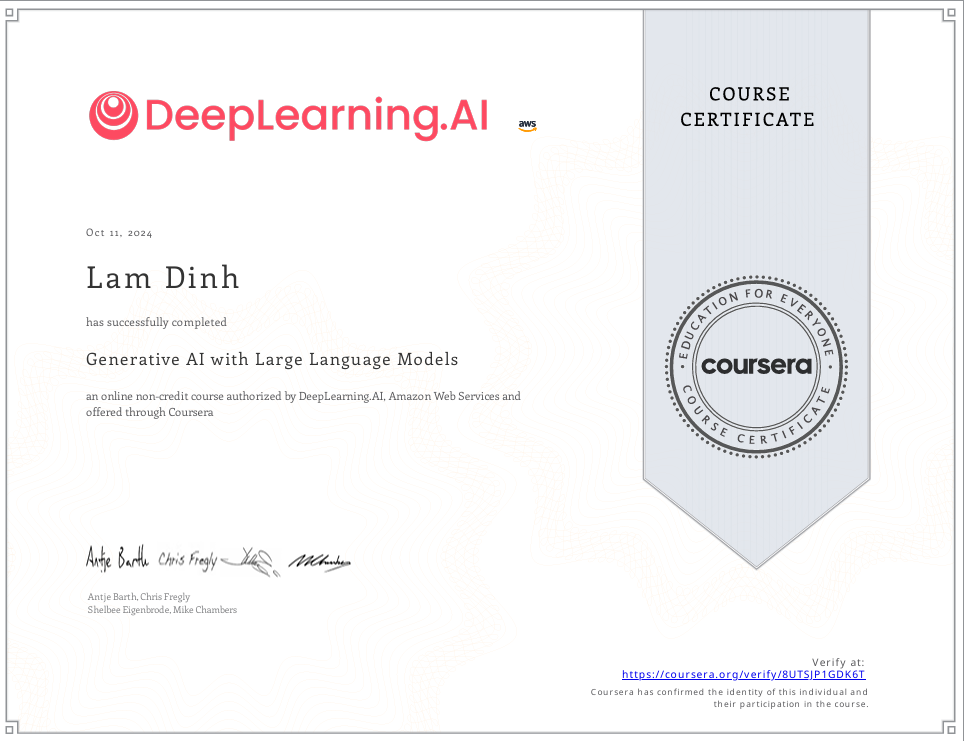
Deep Learning
[C] Neural Networks and Deep Learning
About In this course, we studied the foundational concept of neural networks and deep learning. By the end, we was familiar with the significant technological trends driving the rise of deep learning; build, train, and apply fully connected deep neural networks; implement efficient (vectorized) neural networks; identify key parameters in a neural network’s architecture; and apply deep learning to your own applications. Certificate
About In this course, we studied the foundational concept of neural networks and deep learning. By the end, we was familiar with the significant technological trends driving the rise of deep learning; build, train, and apply fully connected deep neural networks; implement efficient (vectorized) neural networks; identify key parameters in a neural network’s architecture; and apply deep learning to your own applications. Certificate
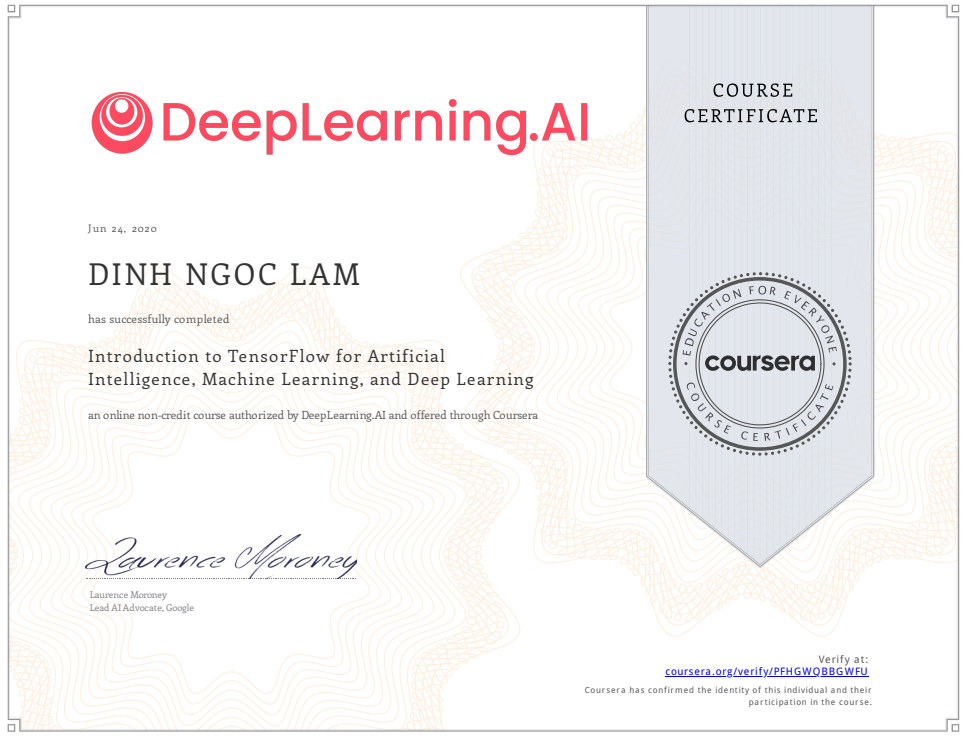
[C] Improving Deep Neural Networks: Hyperparameter Tuning, Regularization and Optimization
About In the second course of the Deep Learning Specialization, we opened the deep learning black box to understand the processes that drive performance and generate good results systematically. By the end, we learned the best practices to train and develop test sets and analyze bias/variance for building deep learning applications; be able to use standard neural network techniques such as initialization, L2 and dropout regularization, hyperparameter tuning, batch normalization, and gradient checking; implement and apply a variety of optimization algorithms, such as mini-batch gradient descent, Momentum, RMSprop and Adam, and check for their convergence; and implement a neural network in TensorFlow. Certificate
About In the second course of the Deep Learning Specialization, we opened the deep learning black box to understand the processes that drive performance and generate good results systematically. By the end, we learned the best practices to train and develop test sets and analyze bias/variance for building deep learning applications; be able to use standard neural network techniques such as initialization, L2 and dropout regularization, hyperparameter tuning, batch normalization, and gradient checking; implement and apply a variety of optimization algorithms, such as mini-batch gradient descent, Momentum, RMSprop and Adam, and check for their convergence; and implement a neural network in TensorFlow. Certificate
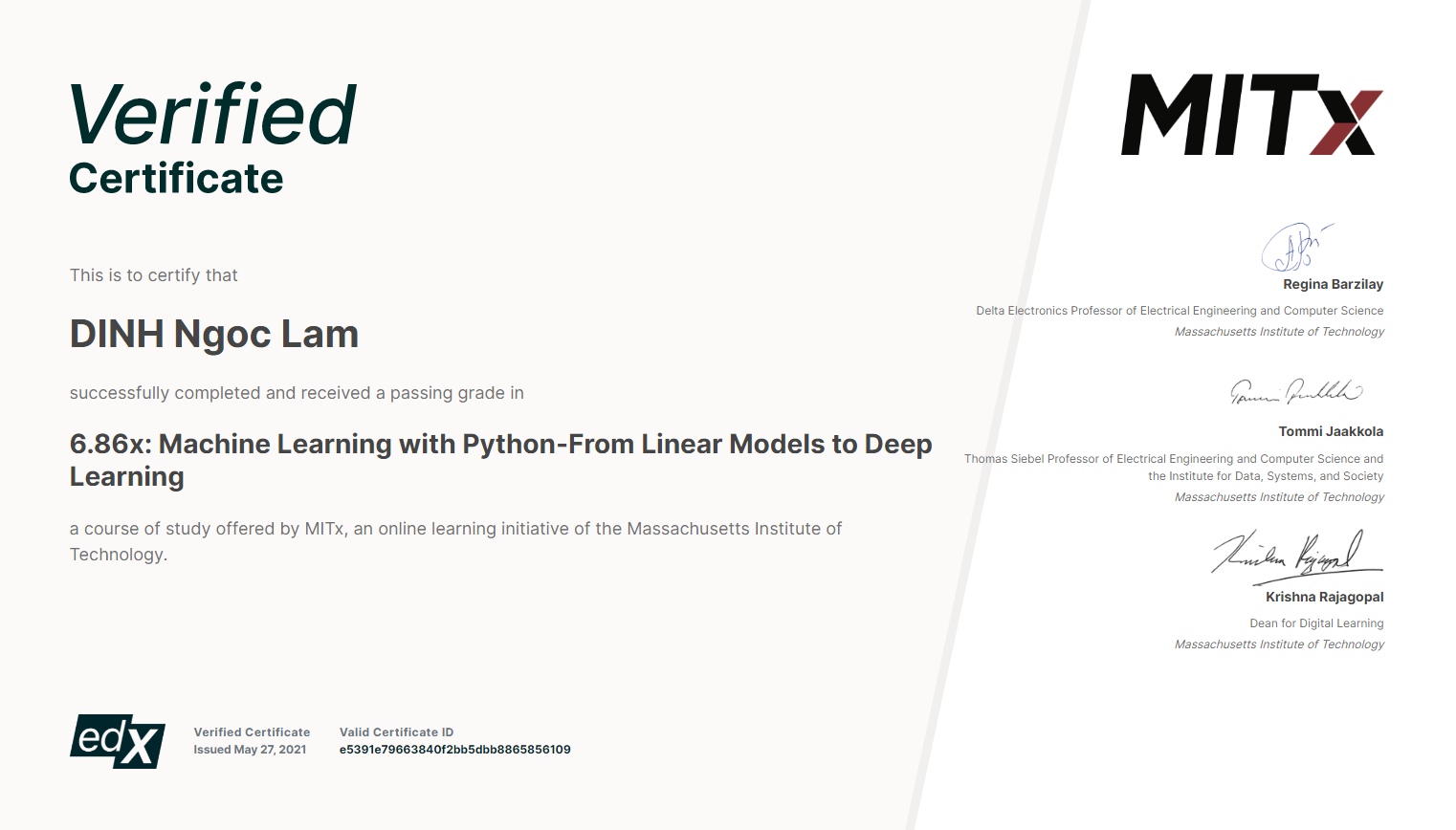
[C] Machine Learning with Python - From Linear Models to Deep Learning
About In this course, we learned: (1) the principles behind machine learning problems such as classification, regression, clustering, and reinforcement learning, (2) Implement and analyze models such as linear models, kernel machines, neural networks, and graphical models, (3) Choose suitable models for different applications and (4) Implement and organize machine learning projects, from training, validation, parameter tuning, to feature engineering. Certificate
About In this course, we learned: (1) the principles behind machine learning problems such as classification, regression, clustering, and reinforcement learning, (2) Implement and analyze models such as linear models, kernel machines, neural networks, and graphical models, (3) Choose suitable models for different applications and (4) Implement and organize machine learning projects, from training, validation, parameter tuning, to feature engineering. Certificate
Reinforcement Learning
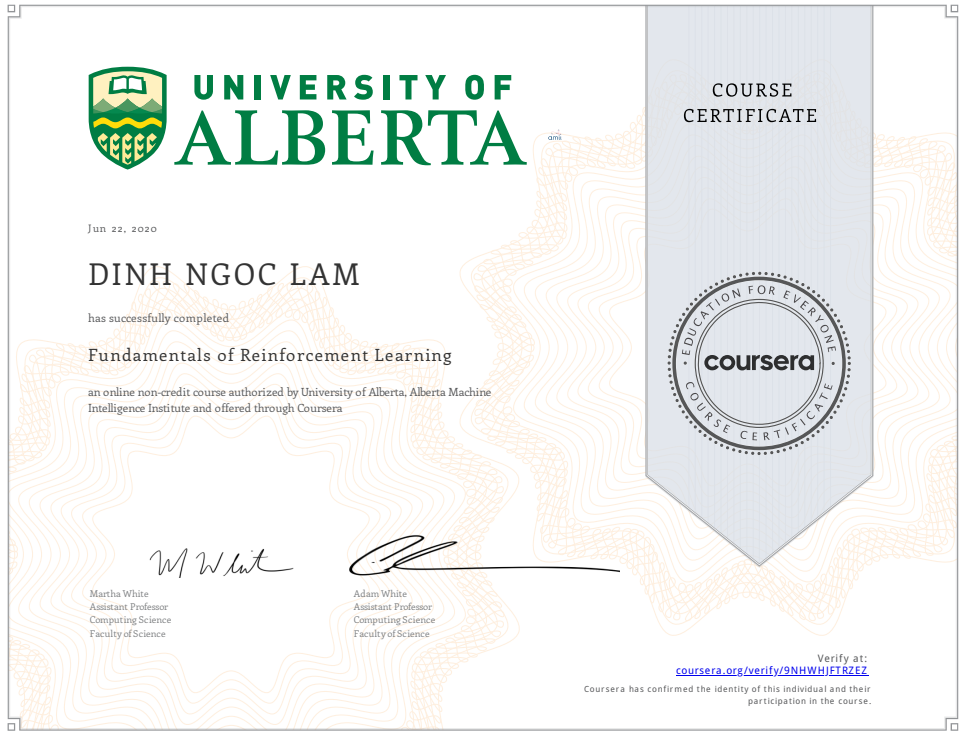
[C] Reinforcement Learning
About This course introduced the fundamentals of Reinforcement Learning including: (1) Formalize problems as Markov Decision Processes, (2) Understand basic exploration methods and the exploration/exploitation tradeoff, (3) Understand value functions, as a general-purpose tool for optimal decision-making and (4) Know how to implement dynamic programming as an efficient solution approach to an industrial control problem. Certificate
About This course introduced the fundamentals of Reinforcement Learning including: (1) Formalize problems as Markov Decision Processes, (2) Understand basic exploration methods and the exploration/exploitation tradeoff, (3) Understand value functions, as a general-purpose tool for optimal decision-making and (4) Know how to implement dynamic programming as an efficient solution approach to an industrial control problem. Certificate
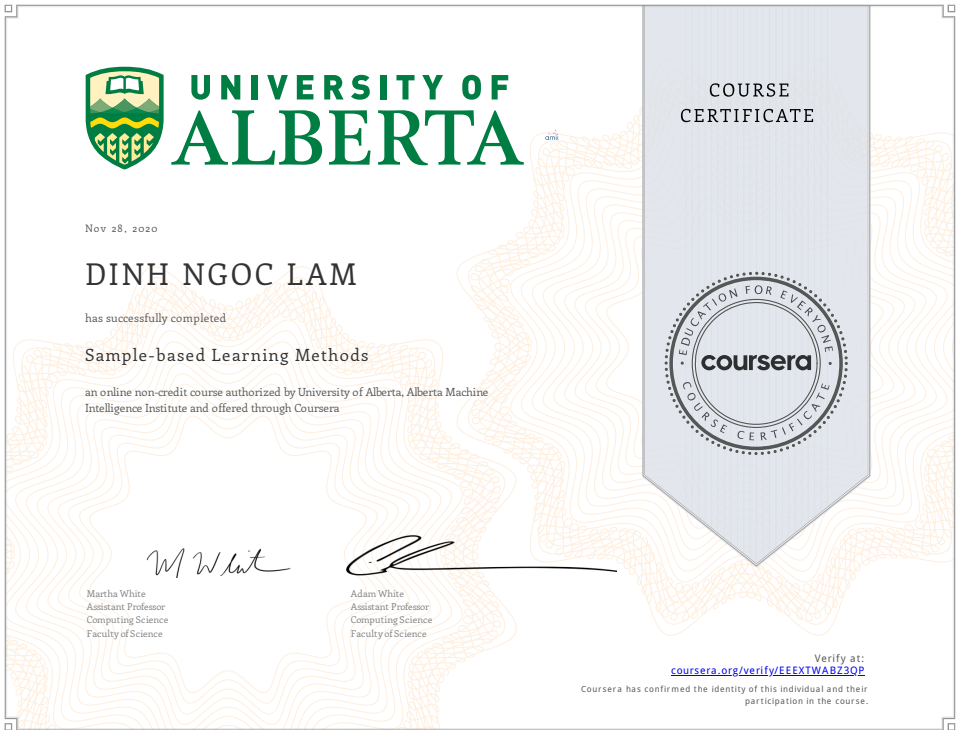
[C] Sample-based Learning Methods
About In this course, we shed light on several algorithms that can learn near optimal policies based on trial and error interaction with the environment. Learning from actual experience is striking because it requires no prior knowledge of the environment’s dynamics, yet can still attain optimal behavior. We covered intuitively simple but powerful Monte Carlo methods, and temporal difference learning methods including Q-learning. We wrapped up this course investigating how we can get the best of both worlds: algorithms that can combine model-based planning (similar to dynamic programming) and temporal difference updates to radically accelerate learning. Certificate
About In this course, we shed light on several algorithms that can learn near optimal policies based on trial and error interaction with the environment. Learning from actual experience is striking because it requires no prior knowledge of the environment’s dynamics, yet can still attain optimal behavior. We covered intuitively simple but powerful Monte Carlo methods, and temporal difference learning methods including Q-learning. We wrapped up this course investigating how we can get the best of both worlds: algorithms that can combine model-based planning (similar to dynamic programming) and temporal difference updates to radically accelerate learning. Certificate
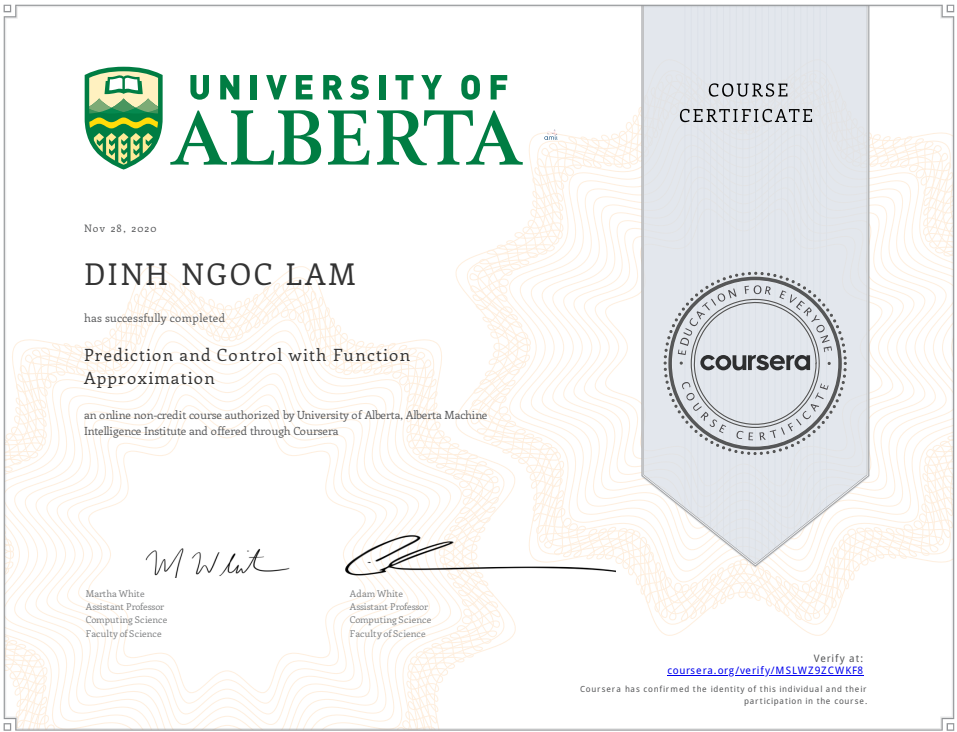
[C] Prediction and Control with Function Approximation
About In this course, we learned how to solve problems with large, high-dimensional, and potentially infinite state spaces. We studied that estimating value functions can be cast as a supervised learning problem (function approximation) allowing you to build agents that carefully balance generalization and discrimination in order to maximize reward. We begun this journey by investigating how our policy evaluation or prediction methods like Monte Carlo and TD can be extended to the function approximation setting. We learned about feature construction techniques for RL, and representation learning via neural networks and backprop. We concluded this course with a deep-dive into policy gradient methods; a way to learn policies directly without learning a value function. In this course we solved two continuous-state control tasks and investigate the benefits of policy gradient methods in a continuous-action environment. Certificate
About In this course, we learned how to solve problems with large, high-dimensional, and potentially infinite state spaces. We studied that estimating value functions can be cast as a supervised learning problem (function approximation) allowing you to build agents that carefully balance generalization and discrimination in order to maximize reward. We begun this journey by investigating how our policy evaluation or prediction methods like Monte Carlo and TD can be extended to the function approximation setting. We learned about feature construction techniques for RL, and representation learning via neural networks and backprop. We concluded this course with a deep-dive into policy gradient methods; a way to learn policies directly without learning a value function. In this course we solved two continuous-state control tasks and investigate the benefits of policy gradient methods in a continuous-action environment. Certificate